How Mistral AI, an OpenAI competitor, rocketed to $2Bn in <12 months
Today I’m diving deep into Mistral AI, who are making headlines after recently closing their (huge) Series A round. Launched just 7 months ago, they’re disrupting the LLM market. I want to look at how they’re doing it - and how you can take advantage.
This post covers:
- What is Mistral?
- Who’s behind it?
- The timeline: What’s happened to date
- Fundraising
- Product Overview
- A peek inside their seed deck 👀
- Roadmap analysis. Are they achieving what they set out to do?
- 5 big reasons Mistral’s making waves 🌊
- How people actually use Mistral
- Opportunities and how you can take advantage
- What developers think of Mistral
What is Mistral?
A French startup that develops fast, open-source and secure language models. Founded in 2023 by Arthur Mensch, Guillaume Lample, and Timothée Lacroix.
They’ve raised over $650M in funding, are valued at $2Bn, are less than a year old and have 22 employees.
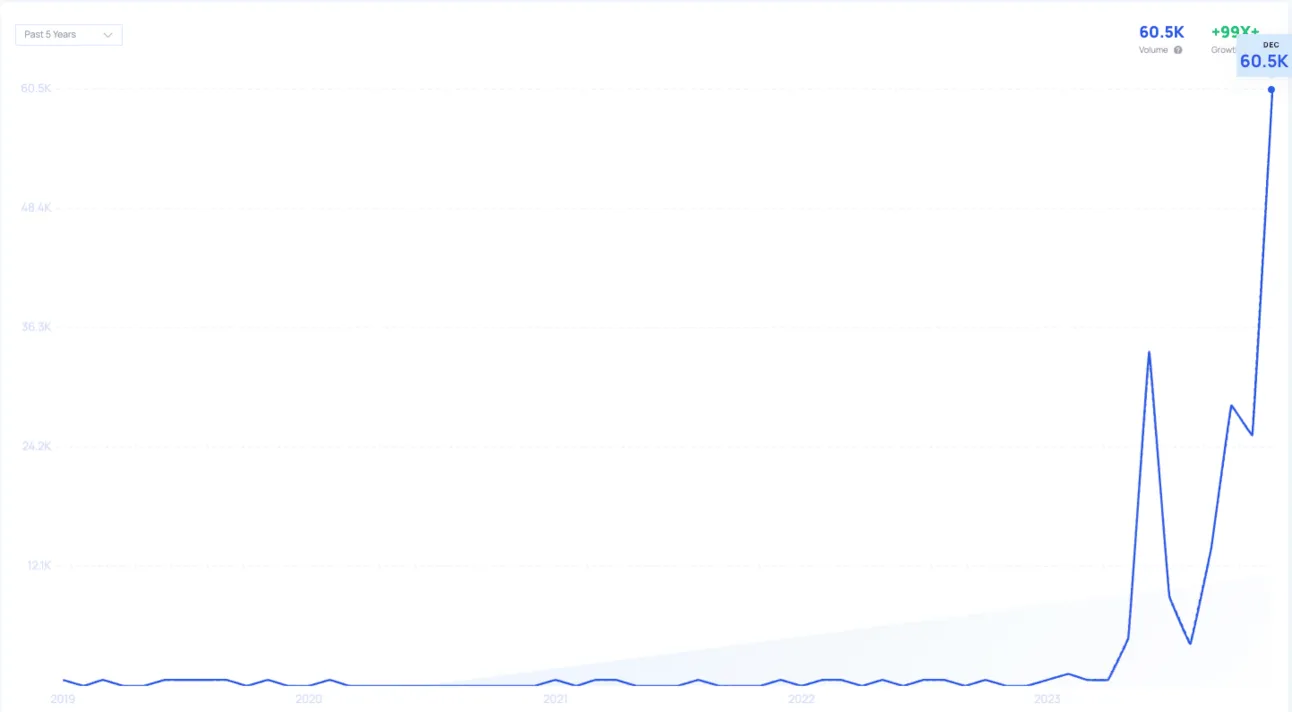
monthly search volume for ‘mistral ai’
The company is important for a few reasons;
- It’s actually open-source, you know like OpenAI was supposed to be? Or how LlaMA by Meta kinda is but isn’t?
- It’s developed 2 AI models in less than a year.
- It’s French.
The founders are 3 researchers from DeepMind and Meta who aimed to beat GPT 3.5 by year-end. And they did.
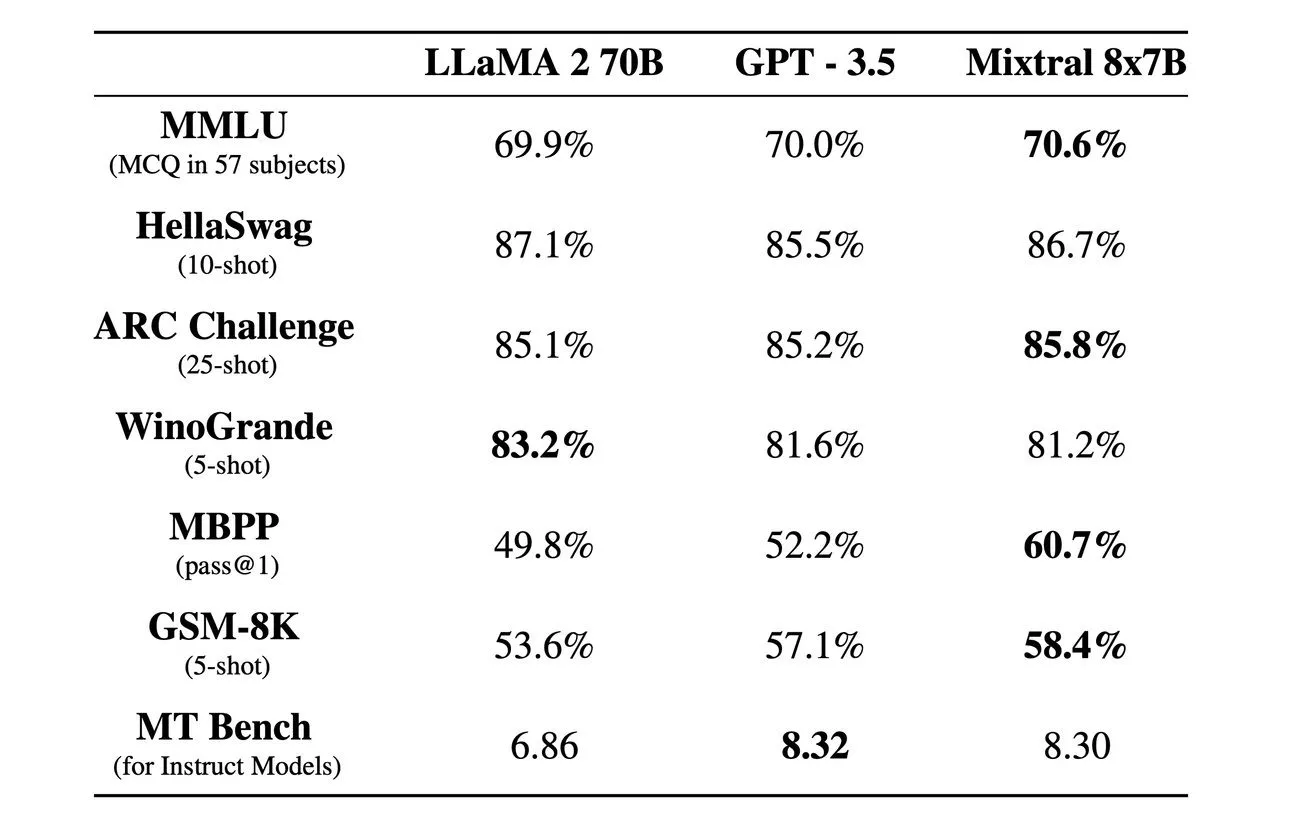
They started a new company, Mistral AI, in May 2023 and had the biggest seed round in the EU within 4 weeks.
Who’s behind it?
Mistral’s CEO Arthur Mensch was at Deepmind for a little less than 3 years where he worked on research around the retrieval-based models, sparse mixture of experts and then co-authored the famous Chinchilla paper on the scaling laws of LLMs.
So he’s legit.
CTO Timothée Lacroix and Chief Scientist Guillaume Lample were at Meta. They both have nearly a decade of experience in research. And, they had just been part of the team behind Meta’s own LLM, LLaMA in February.
Also legit.
The timeline
Here’s a quick rundown of what’s happened since then:
- June 13 2023 - Seed Funding of $113M.
- Sept 27 2023 - Their first model Mistral 7B released (via a torrent link on Twitter X).
- Dec 8 2023 - Mixtral 8x7B MoE released—their second model, again released via a torrent link.
- Dec 11 2023 - Launch of its API and developer platform. Followed by the news of its Series A ($415M) plus debt financing ($130M) by NVIDIA and Salesforce.
Let’s take a quick look at those rounds because they are eyewatering…
Fundraising
Mistral’s Seed Round:
The first funding round took place on 13th June 2023. The company raised $113 million, led by Lightspeed Venture Partners.
Other participants included Redpoint, Index Ventures, Xavier Niel, JCDecaux Holding, Rodolphe Saadé, Motier Ventures, La Famiglia, Headline, Exor Ventures, Sofina, First Minute Capital, and LocalGlobe. Notably, French investment bank Bpifrance and former Google CEO Eric Schmidt were also shareholders.
This funding round valued Mistral AI at $260 million.
Mistral’s Series A Round:
The Series A round was announced on 11th December 2023. In this round, Mistral AI raised $415 million, led by Andreessen Horowitz.
Other participants included Lightspeed Venture Partners, Salesforce, BNP Paribas, General Catalyst, Elad Gil, Conviction, and others. Crunchbase also differentiates Nvidia and Salesforce as debt investors with an additional $130M.
This funding round valued the company at approximately $2 billion.
Product Overview
Mistral 7b
A 7B dense transformer, fast-deployed and easily customisable. Small, yet powerful for a variety of use cases. Supports English and code, and an 8k context window.
Mixtral 8x7B MoE
A 7B sparse Mixture-of-Experts model with stronger capabilities than Mistral 7B. Uses 12B active parameters out of 45B total. Supports multiple languages, code and 32k context window.
It comes in 3 versions:
- tiny
- small
- medium
Embedding
State-of-the-art semantic embeddings from text chunks. Powers your RAG application.
Generation
Efficient chat-based API for text generation, using our open and optimised models under the hood.
You can play with it on; Together’s Playground, Perplexity, Vercel, Langchain’s Langsmith and Hugging Face.
To use the official API check out their docs, plus available on Together, Anyscale, Replicate, Perplexity and many others.
A peek inside their seed deck 👀
Their seed deck has been floating around the internet. Which you can view here.
And there are a few things to mention specifically.
They believe the most value is in the hard-to-make tech e.g. the models themselves. Trained on powerful machines, trillions of words, high-quality sources—which is one barrier to entry.
The other barrier? A talented (and capable) team.
There were a few others on the team at the time of the first raise:
- Jean-Charles Samuelian - CEO of Alan (looks like he is a Co-Founding advisor & Board Member at Mistral)
- Charles Gorintin - CTO of Alan (also Co-Founding Advisor at Mistral)
- Cédric O - Former French Secretary of State for Digital Affairs (also Co-Founding Advisor at Mistral)
Continuing through their deck…
“All major actors are US-based”.
The Mistral team wanted to cement itself as the European leader.
Closed-source vs open-source. The big debate.
Mistral believes (as do many others, myself included) that there are several concerns with closed AI approaches; businesses have to send sensitive data to it, only exposing the outputs doesn’t help connect with other components (retrieval, structure inputs etc) and the data used to train the models are secret (so we assume it can do some things it perhaps hasn’t been trained on).
Now the bold stuff.
“Mistral will offer the best technology in 4 years”.
How?
- They’ll take a more open approach to model development.
- Tighter integration with customers’ workflows.
- Increase focus on data sources and control.
- Propose unmatched guarantees on security and privacy.
There’s a lot more detail in their deck on the above 4 points.
As far as business focus goes…
“On the business side, we will provide the most valuable technology brick to the emerging AI-as-a-service industry that will revolutionise business workflows with generative AI. We will co-build integrated solutions with European integrators and industry clients, and get extremely valuable feedback from this to become the main tool for all companies wanting to leverage AI in Europe.”
Roadmap analysis
Let’s look at their roadmap (remember this was from pre-June) and see what they planned on doing compared to what has happened.
“We will train two generations of models, while developing business integration in parallel.
The first will be partially open-source and rely on tech mastered by the team. It will validate our competence near clients, investors and institutions.
The second will address significant shortcomings of current models to become safely and affordably usable by businesses.”
They’ve achieved most of that:
Mistral 7b ✅
Mixtral 8x7B MoE ✅
But “safe” is hard to define, and I haven’t found much on where they talk about it. And others have found ways to prompt-hack it already (more on that below).
“At the end of 2023 we will train a family of text-generating models that can beat ChatGPT 3.5 and Bard March 2023 by a large margin, as well as open source solutions.”
Mixtral MoE (launched December 2023 ✅) does beat GPT 3.5. Not by a ‘large’ margin, but a beat is a beat.
“Part of this family will be open-sourced; we will engage the community to build on top of it and make it the open standard.”
They released it as a torrent link ✅. And have since changed their Terms of Service so that you can use the outputs to train and/or improve your software (you just can’t use it to reverse engineer the services) - more on that below.
“We will service those models with the same endpoints as our competitor for a fee to acquire third-party usage data, and create a few free consumer interfaces for trademark construction and first-party usage data.”
Is a ChatGPT competitor on the way? I assume that’s what they mean by ‘free consumer interfaces’. They released their API platform which developers are getting access to already. ✅
“In the following six months, these models will be accompanied by semantic embedding models for content search, and multimodal plugins for handling visual inputs.”
So Q4 2023 - Q1 2024. Embeddings are already available in the API. ✅
If their roadmap remains true, here’s what’s in store for next year…
Q1-Q2 2024:
“We will focus on two major aspects that have been under-estimated by incumbent companies:
Train a model small enough to run on a 16GB laptop, while being a helpful AI assistant.
Train models with hot-pluggable extra-context, ranging in the millions of extra words, effectively merging language models and retriever systems.”
At the end of Q2 2024:
“We intend to
- be distributing the best open-source text-generative model, with text and visual output
- own generalist and specialist models with one the highest value/cost
- have scalable and usable and varied APIs for serving our models to third-party integrators
- have privileged commercial relations with one or two major industrial actors committed in using our technology”
And after that:
“Our purpose in the first year is to demonstrate that we are one the best teams of the world in the AI race, able to ship models and model affordances that rival the largest actors.”
“One of the North stars of mistral.ai will be safety: we will release models in a well-staged way, making sure that our models can only be used for purposes aligned with our values—for this, we’ll offer beta access to a “red team” to uncover inappropriate behaviours and correct them.”
“We will thus convince major public and private institutions to trust us for constructing the safe, controllable and efficient technology that we need to make humanity benefit from this science breakthrough — effectively bringing institutions and states for the Serie A round. In that round (Q3 2024), we expect to need to raise 200M, in order to train models exceeding GPT-4 capacities.”
Ok, first thing out of place on the roadmap compared to reality. They already raised their Series A and it was $415M, not $200M. So ❌ I guess? 😅
But given they raised that round already, we can assume a number of these plans are well underway.
5 big reasons Mistral’s making waves 🌊
There are many things to talk about here. So let’s go step-by-step (I’m talking to ChatGPT too much aren’t I?!)
1. Mixtral is a Mixture of Experts model.
A Mixture of Experts (MoE) is a type of model in machine learning that is essentially a team of different models or "experts," each specialised in handling different types of data or tasks.
Imagine you have a group of chefs, where each chef is an expert in cooking a particular type of cuisine. When you want to prepare a meal, you choose the chef whose speciality matches the type of cuisine you want.
Theoretically sounds good. But in practice, some think that…
"due to their high parameter count, more complex architecture and the need to distribute them across multiple GPUs, careful attention must be paid to routing, load balancing, training instabilities and the inference-time provisioning of experts across devices."
https://blog.javid.io/p/mixtures-of-experts
They potentially introduce difficulty from a practical perspective but can gain improvements in certain cases - if you can figure out how to train and use them correctly. As per Cameron’s response to my question.
While others believe...
MoE is the right path forward. It flexibly trades off between knowledge/memorization and efficiency of a small model. OpenAI has been doing this for > 1 yr since GPT-4 training. I'm honestly surprised the AI community didn't route more attention to MoE.
https://x.com/DrJimFan/status/1734269362100437315?s=20
2. It’s open-source.
We touched on closed vs open a bit above:
…there are several concerns with closed AI approaches; businesses have to send sensitive data to it, only exposing the outputs doesn’t help connect with other components (retrieval, structure inputs etc) and the data used to train the models are secret (so we assume it can do some things it perhaps hasn’t been trained on).
When asked about why open-source Arthur’s replies have a consistent line of belief: open source leads to better models.
- By giving to the community, you can take from the community. The community can take open models and make them better for specific purposes.
- We think it’s really damaging to the science to mode into an opaque setting where companies are spending billions of compute doing the exact same thing and not communicating about it.
And we’ve seen a glimpse of it. As Mistral has been true to the promise of releasing open models, the community (and other companies) have taken Mistral’s models and created better models on top of them. For example, OpenHermes 2.5 by Teknium and Neural Chat 7B by Intel.
With AI models, open source takes many forms: from available to use locally but no details about the model (weights, architecture etc.) to models that are fully open source and allow users to train on the outputs.
While Mistral’s models were open-weights from the start, Mistral’s latest announcement had a line in their Terms of Service Terms of Service which was spotted by Far El on Twitter, said:
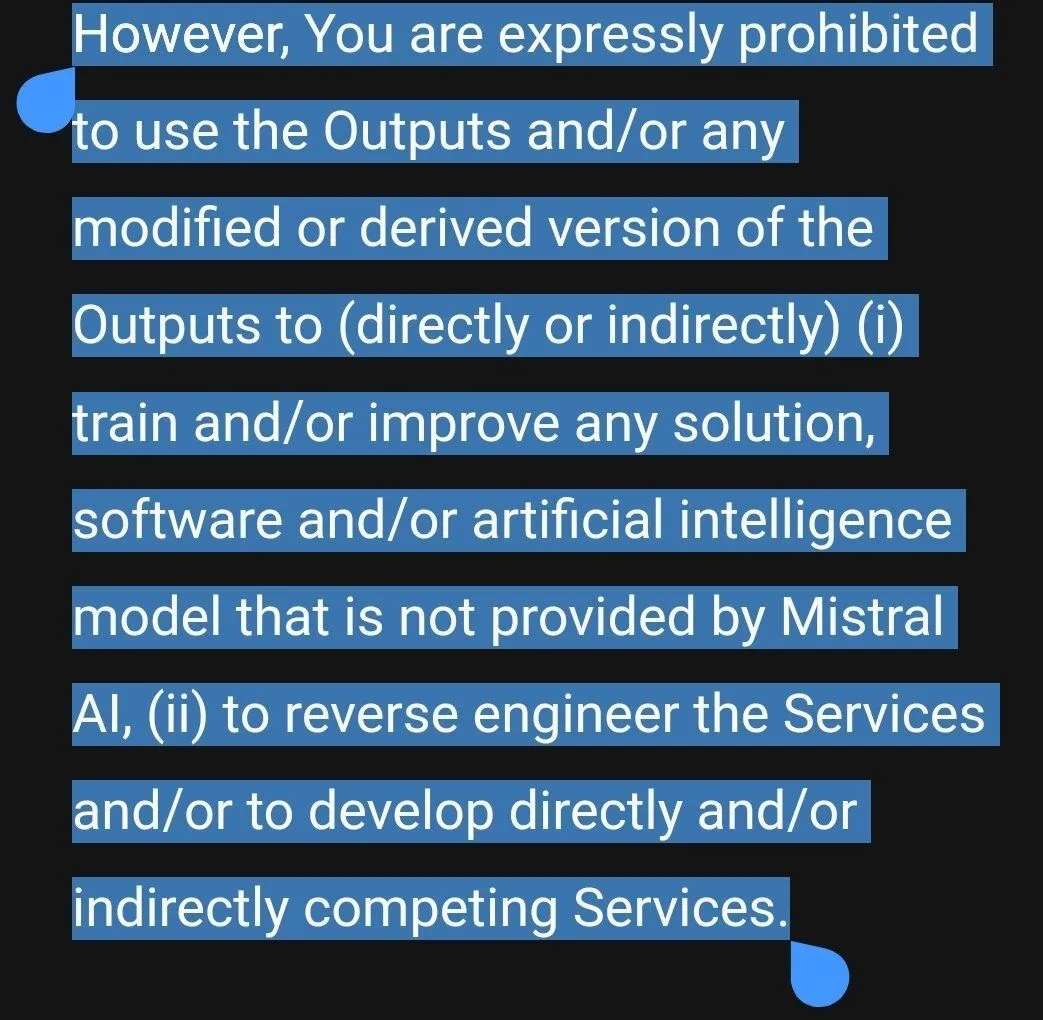
Basically, you can’t use it to train or improve other models or compete against them…
It wasn’t clear whether this was just for the API platform or the model itself. And unfortunately, open-source means you should be able to use this tech how you like, that’s kind of the point. The models are under the Apache 2.0 licence, so you can do what you like. It’s not enforceable.
Here’s the Apache 2.0 definition:
It allows users to use, modify, and distribute the licensed software, including creating derivative works, for any purpose without concern for royalties.
Nevertheless, Arthur, the CEO of Mistral, saw this tweet and swiftly removed that line in the ToS. Announced in a tweet.
The implications of this move are pretty big.
“Allowing us to use the output to train other models is the biggest release. It instantly unleashes the open source community.
Why? The best smaller models today are made using gpt4 outputs, but they're hampered by the openai terms. Mixtral (and their bigger models coming soon) can now be used to generate output to train smaller models. Things will now keep accelerating.”
https://x.com/dctanner/status/1734563550222237956?s=20
(we’ll touch on the bit about being trained on GPT-4 outputs shortly)
A move like this also gains trust and loyalty from the community (look at the sheer number of excited replies to Arthur’s tweet).
“Respect for @arthurmensch and @MistralAI for the quick response. They did what [OpenAI] or Meta haven't had the courage to do (yet). Excited to see what's next!”
The new ToS line was changed to basically say “don’t use it to reverse engineer our services”.
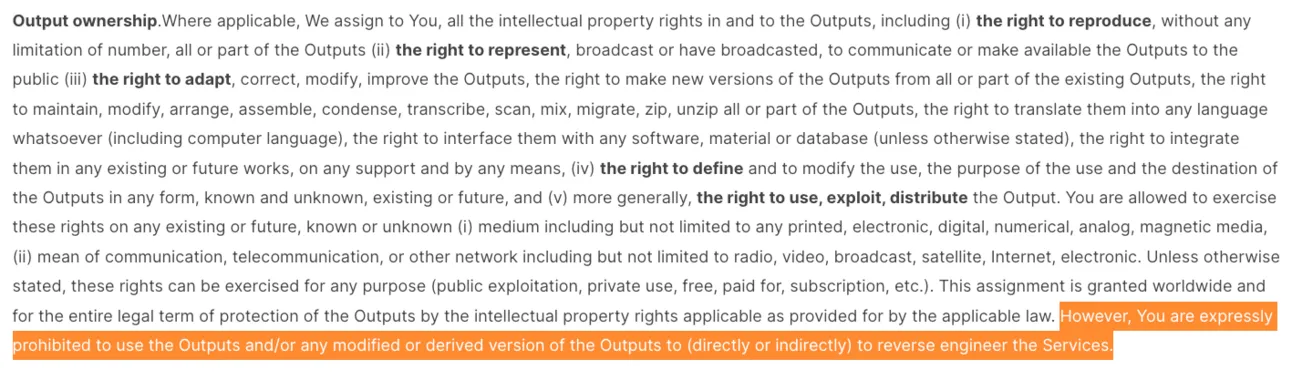
Fair enough. A lot of trust there.
An opposing view on open-source LLMs is:
The point here is really that open-source LLMs will struggle to beat OpenAI at its own game. If you want a global product with the largest reach and best model, then OpenAI looks to be so far ahead. Bard, Pi, and others are also trying to claim the top spot.
But if you only look at it through that lens.
Open-source LLMs maybe don’t need to be competing there. They can be used for many different use cases.
Everything will get cheaper, faster, and more accurate. But open-source LLMs may always stay that much cheaper and faster. And better for specific tasks it’s trained on.
3. It’s cheap.
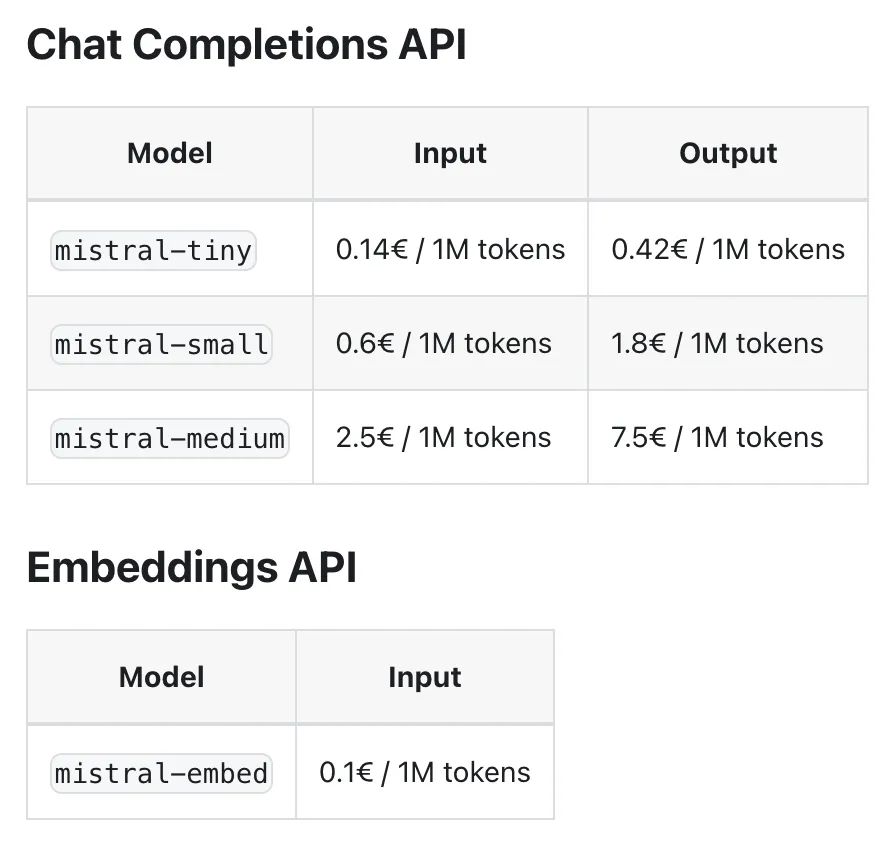
Together AI (a cloud platform for building and running generative AI) seemingly undercut Mistral’s pricing by 70% on their platform.
We have optimized the Together Inference Engine for Mixtral and it is available at up to 100 token/s for $0.0006/1K tokens — to our knowledge the fastest performance at the lowest price!
https://x.com/togethercompute/status/1734282721982324936?s=20
There was a little confusion here given € → $ conversion, and 1k → 1M tokens. 0.6/1M is 0.0006/1k.
So I asked a friend who works at Together…
"Mistral charges $0.65/million tokens for input tokens, but then charges a much higher price of 1.8 euros per million ($1.94) for output tokens, as opposed to Together AI that charges $0.6/million tokens for both. Output tokens can often be much more common than input tokens since it’s normal for your prompt to be much shorter than your output. But even assuming that input and output tokens are equally used, that still leaves Mistral at an average of $1.295/million tokens which is more than 2x more expensive than Together AI at $0.6/million tokens."
friend who works at Together AI
The big reason Together can do this is because of their insane Inference engine. And they don’t have to recoup the training costs taken on by Mistral in the first place (possibly a reason?). The beauty (or beast) of open-source is that Together can reduce the cost if their tech enables it to.
Worth noting here that Mistral is building its own inference engine too.
“The coefficient you put in between the inference cost and training cost is very business dependent and as a company that intends to have a valid business model, we think a lot about inference cost. We knew that we could make a 7B model that is very good and you can run it anywhere.”
No priors podcast
Wait, what’s an inference engine?
It’s a component of an intelligent system that applies logical rules to existing information to deduce new knowledge. From Wikipedia:
“A trivial example: In forward chaining, the inference engine would find any facts in the knowledge base that matched Human(x) and for each fact it found would add the new information Mortal(x) to the knowledge base. So if it found an object called Socrates that was human it would deduce that Socrates was mortal. In backward chaining, the system would be given a goal, e.g. answer the question is Socrates mortal? It would search through the knowledge base and determine if Socrates was human and, if so, would assert he is also mortal.”
While writing this post, many others have come out with undercutting pricing… I rewrote this section every time so here’s the TLDR:
- Mistral: €0.6/1M input tokens & €1.8/1M output tokens
- Together: $0.6/1M tokens (offering $25 credit upfront to new users)
- “If you use that on mixtral 8x7B that's 40 million tokens. That's GPT3.5 capability, probably around 10,000 chatbot interactions (assuming a typical bot conversation is around 4000 tokens).” source.
- Perplexity: $0.14/1M input tokens & $0.56/1M output tokens (if you sign up for Perplexity Pro you get $5/month credit)
- Anyscale: $0.5/1M tokens
LLMs are being commoditised.
4. Safety.
Safety is one of the core things Mistral is thinking about. But it’s not interested in censoring the base model.
"We think the benefits of providing open source models to the community are great for safety because if you have access to weights of the model, you can tweak it to do a moderation model, which counteracts the bad usage of generative AI."
- Via Stripe AI Day Paris
“You don't want to ban outputs from the raw model because if you want to use it for moderation, you want your model to know about this stuff. So, assuming that the model should be well-behaved is I think a wrong assumption. You need to make the assumption that the model should know everything and on top of that you should have some modules that moderate and guardrail the model.”
- Via No Priors Podcast
A portfolio founder/developer told me:
They're not training it to refuse instructions. They're not training it to not get prompt-tacked. They're going to rely on the community for that, and the community is going to build this model into something much better than it is today.
Likely, Mistral would also provide these moderation modules for the application makers.
Mistral is also based in France, and given the EU AI Act (whatever your view on it…) directly impacts them, Arthur has written about Mistral’s position on the act. It reflects this ideology of focusing on product safety, not model censorship.
Another lens to look at safety is if you can prompt the model to say things it shouldn’t. These things in question are often harmful (ranging from jacking a car to creating bioweapons). It feels like there are always going to be examples that prove and dispel this. For example, mistral-instruct has a very censored-ish reply to creating black smoke from car exhaust, while you can easily prompt hack the model.
Arthur mentioned this in a podcast recently:
Knowledge is not the bottleneck to harmful use cases. LLMs are not substantially better compared to search engines in finding harmful knowledge.
These models have access to vast amounts of information, so the issue isn't about what they know or don't know. For certain tasks or applications, LLMs do not offer a major advantage over what you can achieve with a search engine. To deal with hallucination (causing misinformation), again, Arthur highlights the ability to fully fine-tune the models to focus on a given source of knowledge.
5. It’s small.
And that’s good for a few reasons:
- Big models cost a lot to predict every token. Smaller models cost less.
- Big models take longer to run.
- Smaller models fine-tuned on a specific task will potentially have higher accuracy on that task.
- Small models are easier to deploy on things like smartphones.
- Small models consume less energy.
Big models however are better in more use cases and benchmarks and are generally have higher accuracy.
Other small models:
- Microsoft just released a small model: Phi-2
- Together AI in collaboration with just released: Mamba-3B-SlimPJ
- Llama-2 7b is a small model from Meta (will we see Llama-3 before year-end?!)
- Gemini Nano from Google
- Alpaca from Stanford
- Vicuna
- Koala from Berkeley
- Zephyr (fine-tuned version of Mistral)
- Falcon
- the list goes on
There’s a leaderboard of all the models here.
What Developers think
“a very very good model”
It’s a very very good model. It looks to be like a GPT 3.7 level model with fine-tuning, especially full fine tuning (which you can’t do with GPT 3.5). That's obviously not proven, but it's pretty realistic to believe. You'll be able to get better than GPT 4 performance with really, really low inference cost. I mean, this changes a lot. You're not going to get a better chat model than GPT 4, at least, I don't think. But you'll get pretty close, and for actual tasks, this thing probably can beat GPT 4 if you train it on enough data, which is incredible for the cost, the speed, the size, everything.
“It seems like it’s got OpenAI outputs in it’s training data.”
As you can see from the tweet below, when asking “Tell me a joke” it repeats the same thing as OpenAI (every time).
The internet has a lot of Open AI outputs, but it does seem like there were some outputs that clearly stuck into this thing, because it outputs that joke like, "first try" every time. And a few other models do as well. So it's a little interesting to see that.
https://x.com/mattshumer_/status/1734605812054827364?s=20
“I don't think there's any reason to use GPT 3.5 anymore”
The big thing is, for the cost and the speed, the performance is utterly ridiculous, it seems. I don't think there's any reason to use GPT 3.5 anymore, especially once they're hosted, API-compatible versions of this, which if there aren't already, there will be very soon.
“very, very fast to train.”
Training is very fast, way faster than I expected. I've already done a few fine tunes on this thing, and I trained a chat model on a pretty large dataset in a matter of nine hours on 6H100s, which is a pretty hefty setup for the average person, but still, very, very fast to train.
“the first base model that has some element of instruction tuning dataset”
This seems to be the first base model that has some element of instruction tuning dataset in the base model. So typically you train it on a big portion of the internet, no instruction tuning data, and then you take that base model and you fine tune it on instruction tuning data, and then you do RLHF and all this stuff to teach it how to respond like a chatbot. But it seems like, whether this was from just exposure to the internet or intentionally done, this model can sort of act in that way without needing to be instruction tuned. It's not great, but it does seem like some of that data definitely leaked into the training in some way or another.
My thoughts on Mistral's stellar rise:
- Successful setup: founded at good timing in the OSS vs closed AI debate, closed a $400M Series A at $2B valuation, and driven by a lean team.
- There're dozens of models coming out every month, but only a handful actually have staying… twitter.com/i/web/status/1…
— Jim Fan (@DrJimFan)
Dec 11, 2023
A very detailed report on Reddit here.
“on-par or better with GPT-3.5 Turbo”
I think it's just magical that Model of Experts (MoE) works really well, the speed and cost as a 12.9B equivalent model that performs on-par or better with GPT-3.5 Turbo (rumors said it's a 20B model).
How Mixtral works is that for every next-token prediction, it only utilized 2 experts from the 8 experts (46.7B total parameters) through routers. But MoE is not really good for local inference as it's using quite a lot of RAM (for example, it uses 100GB RAM on 16-bit precision on Apple Silicon), I think there will be a lot of new research or projects coming out soon around fine-tuning MoE, or memory-efficient local inference techniques...
The next goal should be having a local GPT-3.5 that can run on the laptop or even mobile device, which is where Phi-2 seems really promising (maybe there could even be a Phi-2 MoE!).
Another thing is MoE is quite cost-effective to train, Mistral reuse the model weights from Mistral-7B for all of their experts.
Opportunities and how you can take advantage
How people are using Mistral
LLMs are generally good at the same kinds of things but having a small model could be more beneficial if you want to create:
- customised AI assistants across specific industries
- multilingual content and translation
- specialised learning platforms with adaptive learning paths
- internal company operations
With new platforms come new ecosystem interest. You could create an expert job board for developers who are great at using and fine-tuning small models, a community of people building with Mixtral and other small models, a course or a YouTube channel teaching people how to use them.
Other ideas can be seen when you look at what large companies are doing (and paying for). And seeing how the same would apply to smaller companies without the same budget.
A report by CBInsights shows the cost spent on LLMs from company execs.
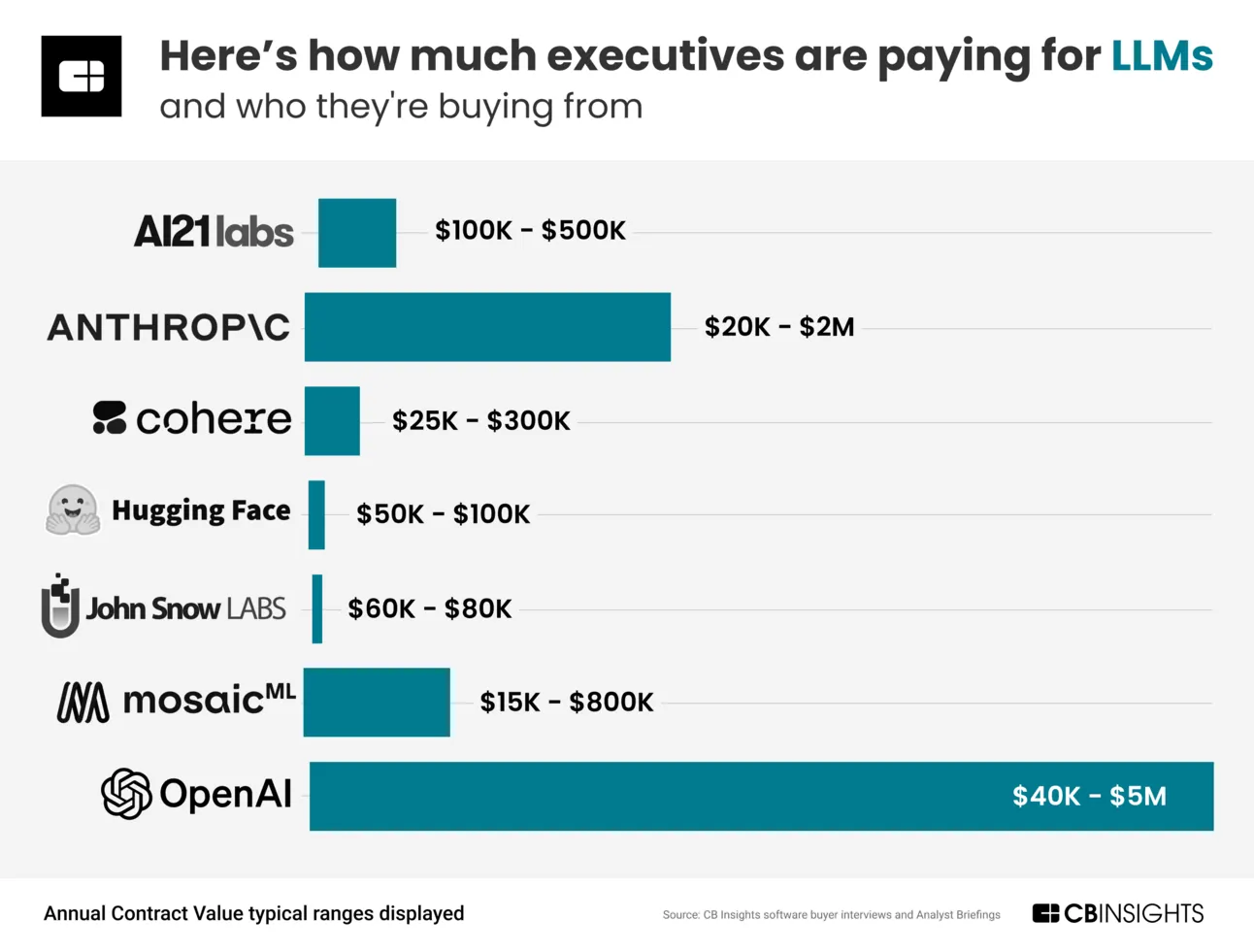
They conducted interviews on this spending and found:
- There’s no clear winner yet in foundation models: Despite OpenAI’s dominance, buyers are still closely evaluating other model developers against key criteria like latency, ethical response, and token limits before making purchasing decisions.
- Open-source models are competing with proprietary models on price: Some buyers have indicated that open-source models like Meta’s Llama2 have comparable performance and lower deployment costs, resulting in better ROI for the organization.
- Domain-specific models are emerging to compete with general-purpose models: Buyers are considering models trained on industry-specific datasets like clinical notes and financial data for domain-specific tasks as an alternative to models trained on general text.
People want it cheap and accurate. Get those things nailed and you’re 80/20 on what the buyers want.
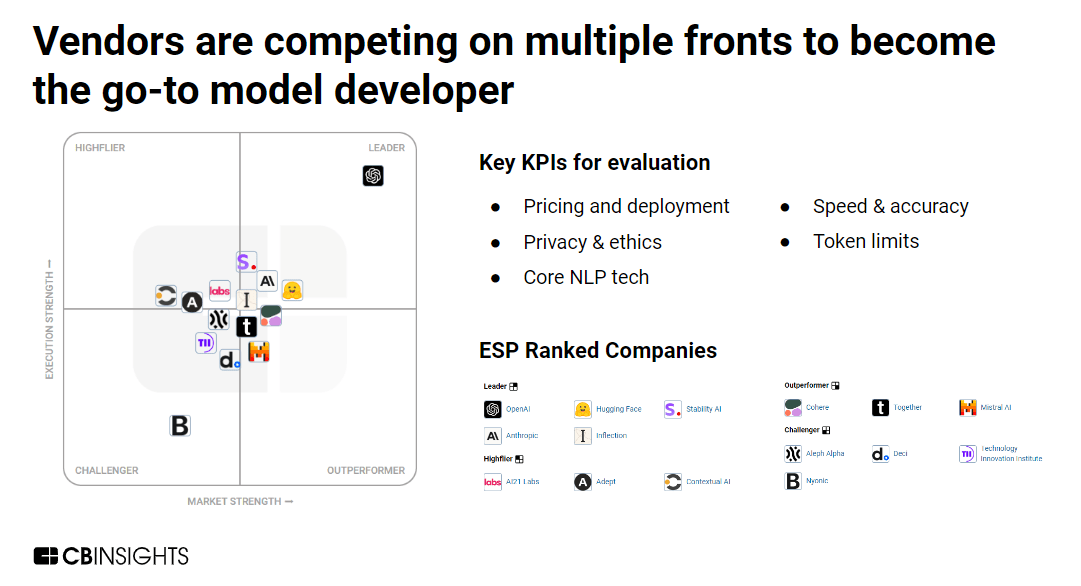
There’s a big opportunity here to bridge the gap.
If you think of all the ways LLMs can be useful internally at companies (querying how many customers signed up yesterday, what is our Christmas break policy, etc) then using an open-source model that doesn’t get your data, is super-cheap to run and is fast, could be the best solution.
Many people use OpenAI as it’s the leader, has integrations with many providers, great documentation etc. People would use these other models (for all the reasons we’ve described) if it were only simple enough to deploy.
Enterprises may require using Azure - Mistral (and other small models) are available there.
“…Meta’s invention… it may be cheaper than the OpenAI [model] because it’s open source. Then we believe the performance of the Hugging Face and also the Llama-2 is also comparable to the OpenAI [model]. Maybe just a little bit weaker than that, but maybe the overall…ROI is quite a good deal.”
— Cloud Data & AI Lead, Fortune 500 company
A Fortune 500 company would likely be open to switching if the performance of open-source models was good enough.
“For the vendor to get to a 9.5 or 10 satisfaction score, they would need to continue to compete on cost level.
C-level Executive, VC-backed entertainment company
Open models are much more cost-effective.
“Strengths, I would say, the ethical considerations of privacy and bias, fairness… their model outperformed the other models, including GPT-3 and ChatGPT… In terms of weaknesses, the specificity of the model output and the interestingness of the model output… I think that other weakness also was in terms of speed and efficiency, like latency, and once you ask a question, how long does it take to fully respond.”
— Senior Manager of Data Science, $1B+ valuation technology company
Buyers are looking at safety concerns as a reason to use a model. If Mistral hits its promise of safety, it could be a great alternative to other bigger, more expensive and slower models.
More like this
Start learning today
If you scrolled this far, you must be a little interested...
Start learning ->Join 3,107 professionals already learning
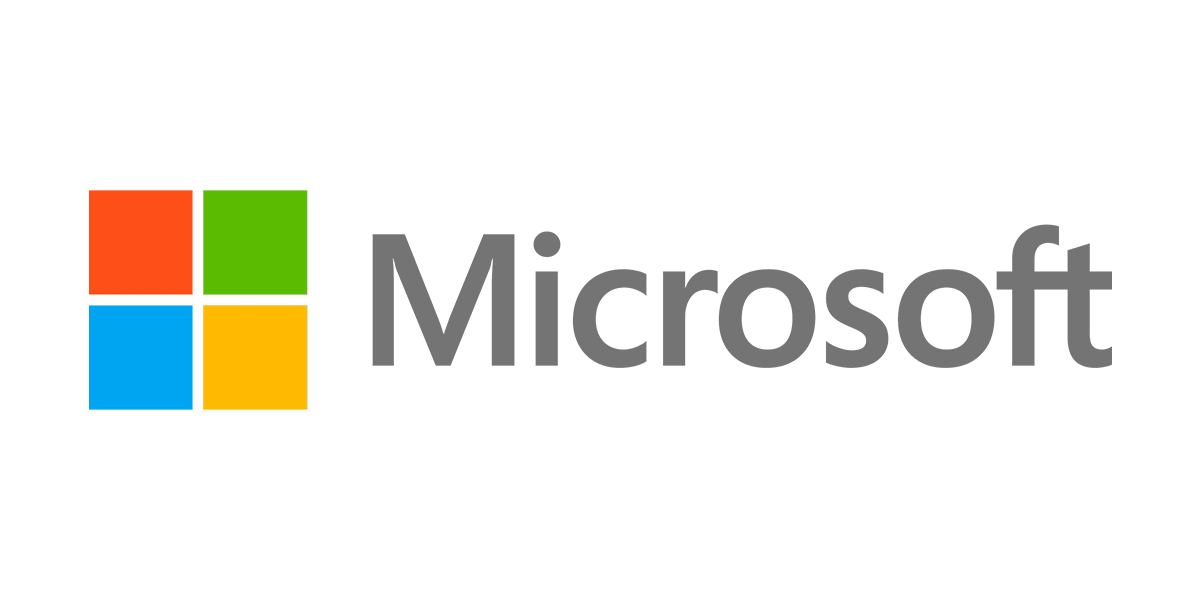
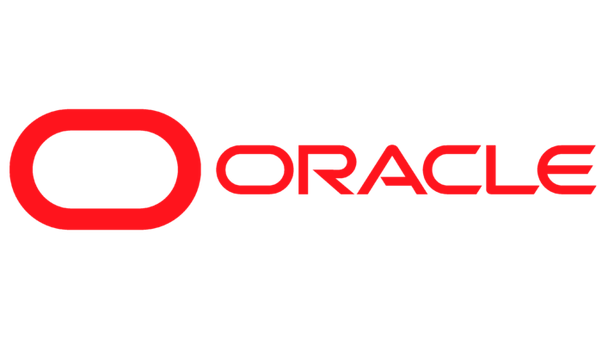
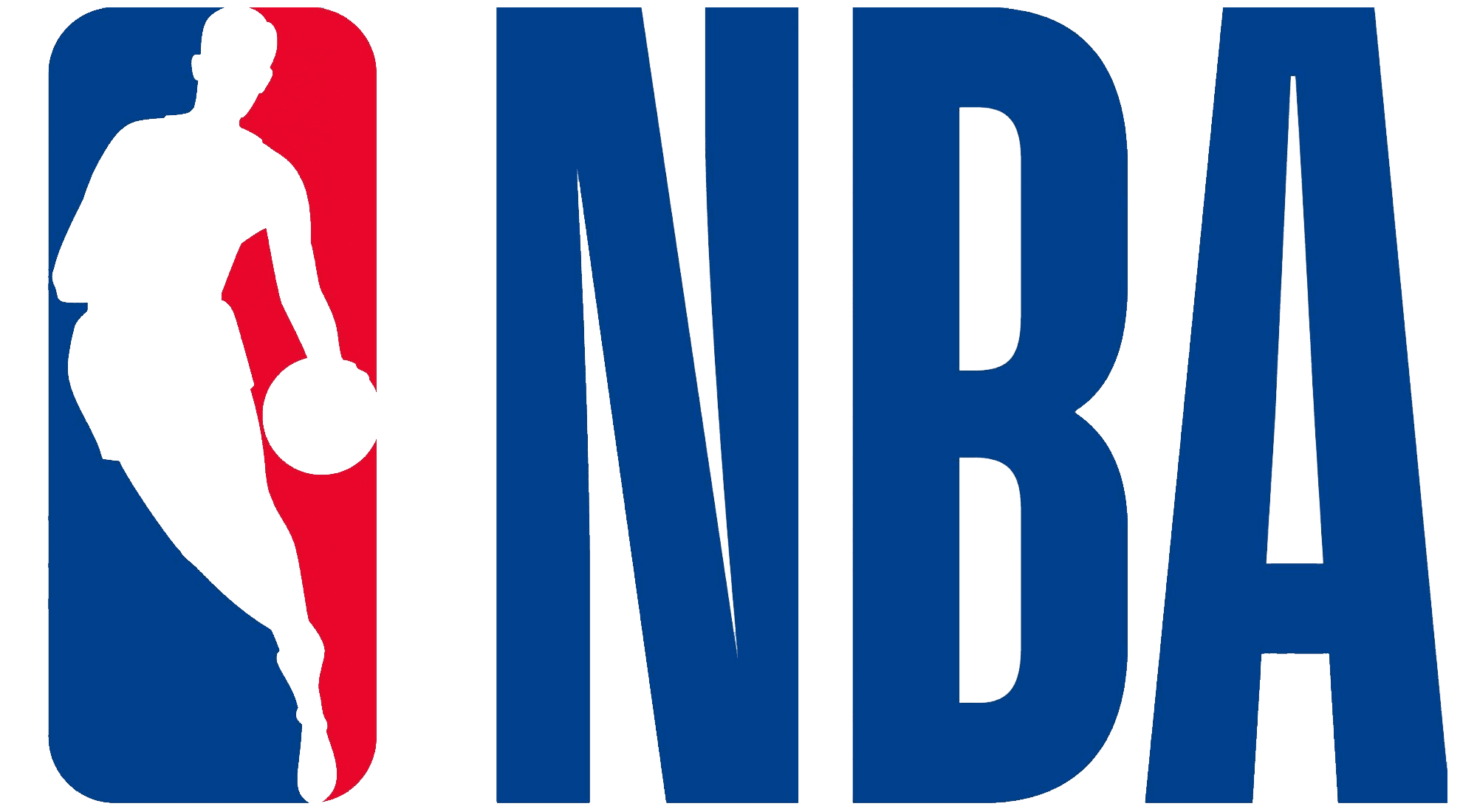
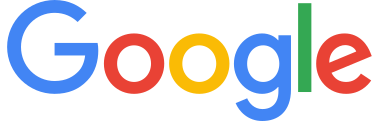
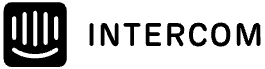
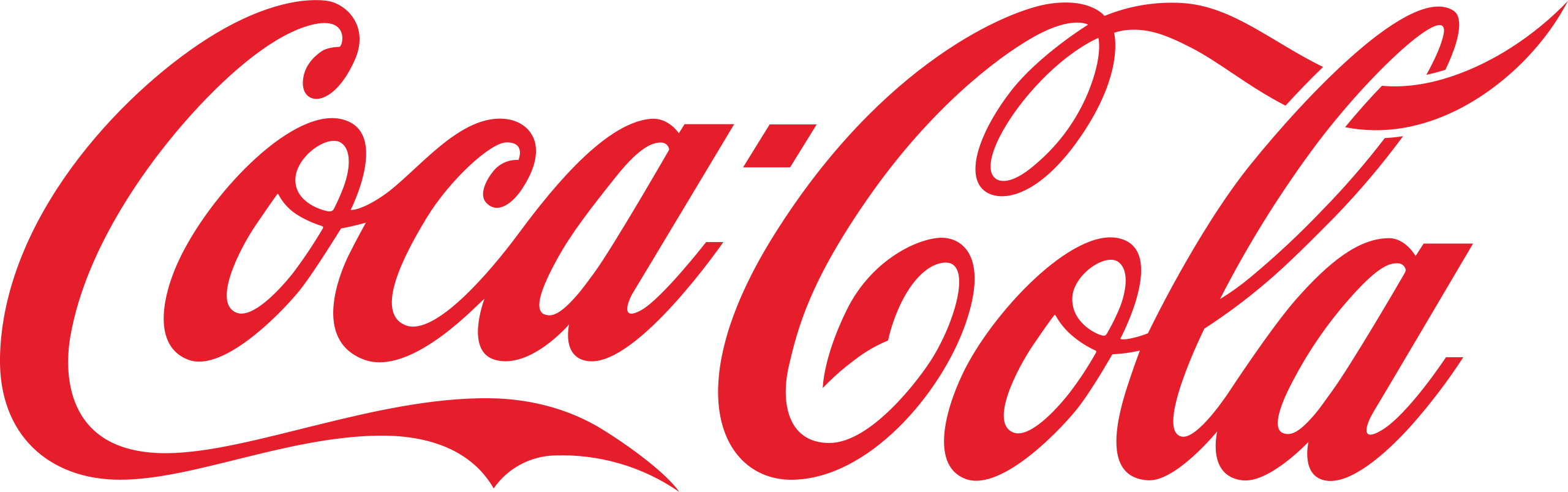
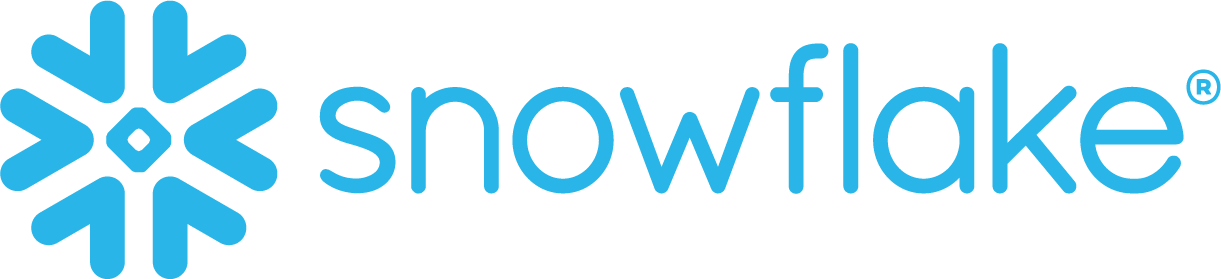
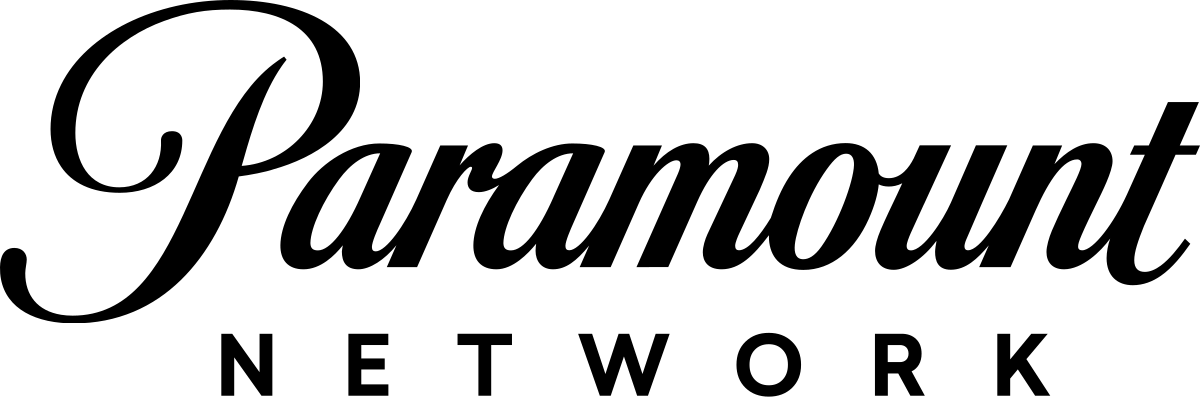
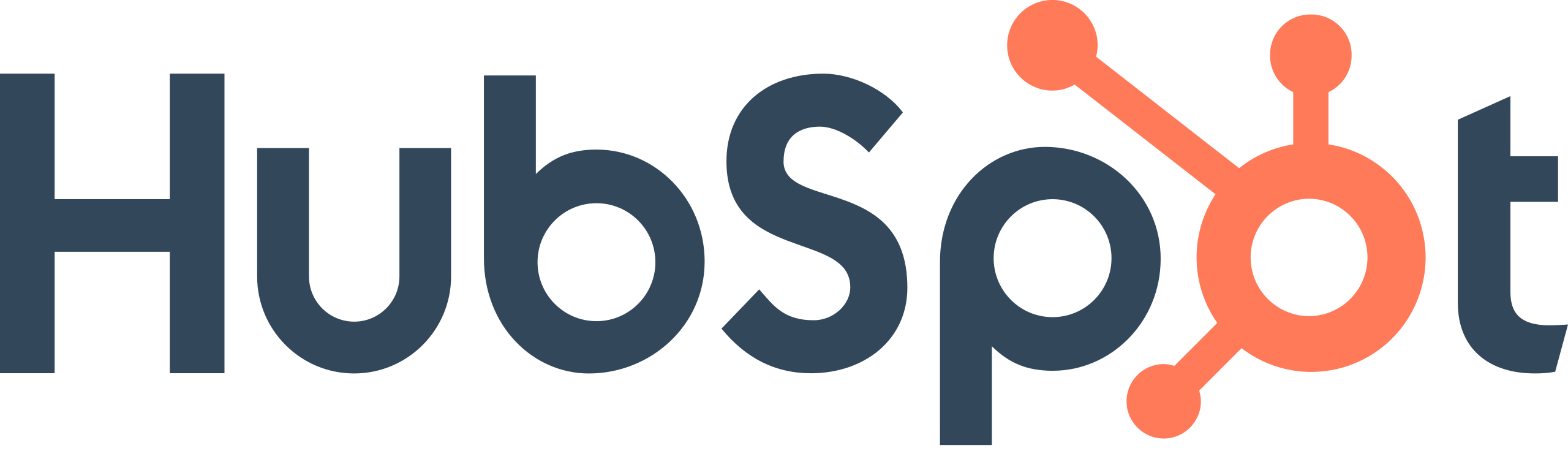
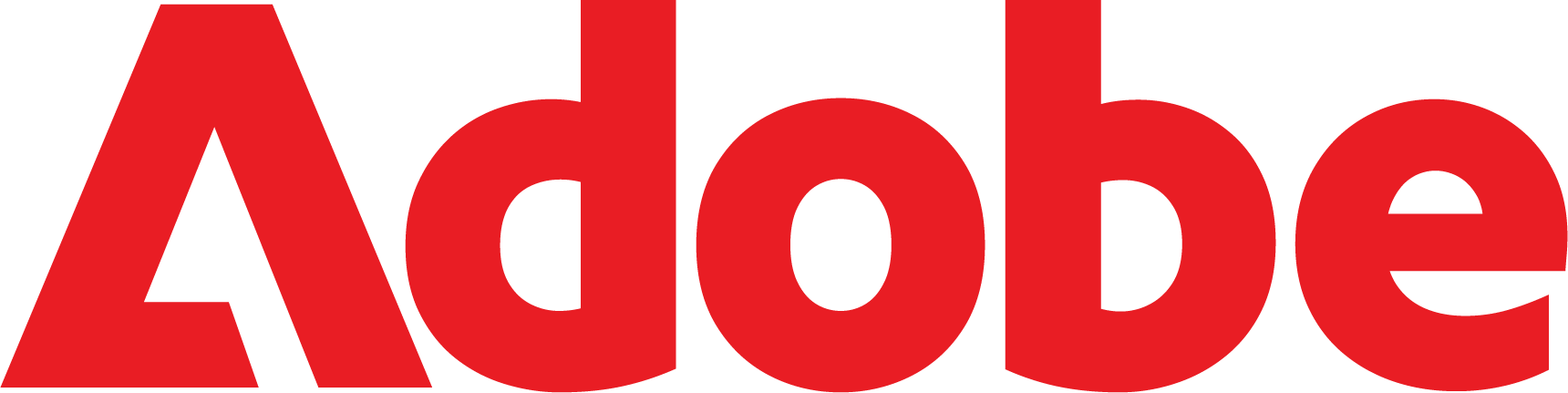
.png)
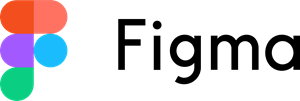